What is a data governance framework? Guide with template
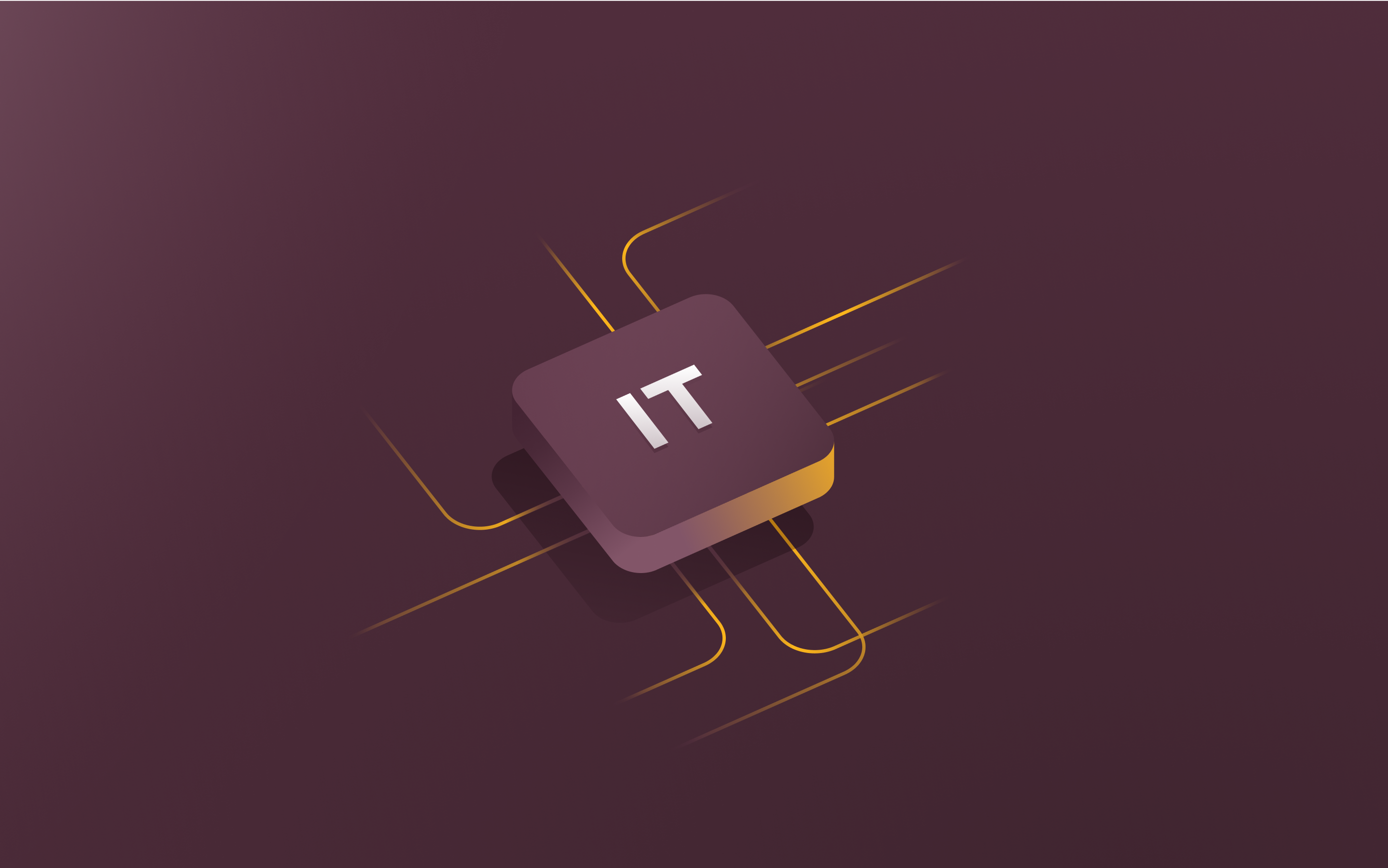
Have you ever been in a meeting where two people present conflicting data, leading to confusion and doubt? Or have you struggled to find the data you need because it's scattered across different systems and departments? These are the problems that a data governance framework is designed to solve.
By providing clear guidelines and accountability for data management, a governance framework helps ensure that an organization's data is consistent, trustworthy, and accessible. And in this guide, we'll explore exactly how it works.
What is a data governance framework?
A data governance framework sets up the rules and processes for how an organization manages its data—from who can access it, to how it's protected, to making sure it meets quality standards and follows regulations. But it goes beyond just managing data. It's also about making sure your data efforts actually help achieve what your organization needs to accomplish, while protecting against risks along the way.
Think of it as a playbook that everyone in your organization follows when it comes to handling data. It defines who's responsible for what data (data owners and stewards), what standards the data needs to meet (data quality), how it's protected (data security), and how it complies with regulations (data compliance).
The goal of a data governance framework is to treat data as a strategic asset. By putting clear guidelines and accountability in place, you can ensure that your data is reliable, secure, and driving value for your business.
How do data governance frameworks work?
The success of a data governance framework depends on three key elements working together seamlessly: people, processes, and technology. Let's break down how this actually works in practice.
First, the framework establishes clear roles and responsibilities within your organization. In other words, making sure someone is actually responsible for keeping your data in good shape. Most organizations have data owners (senior leaders who are accountable for specific types of data), data stewards (who handle day-to-day data quality and governance), and data custodians (who manage the technical side, typically IT teams). These roles might look a bit different depending on your organization's structure, but the key is having clear ownership at every level.
But having roles isn't enough. Your framework also needs to spell out exactly how data should be handled throughout its lifecycle. This means creating clear processes for how data gets collected, stored, used, shared, and eventually archived or deleted. These processes shouldn't be overly complex, they just need to be clear enough that everyone knows what to do.
Finally, you need the right technology to support all this work. While you can start by making better use of your existing tools, growing data volumes often call for specialized solutions that can automate and enforce your governance processes at scale. The key is choosing tools that match your organization's needs and can grow with you—whether that's better utilizing what you have or bringing in new systems designed specifically for data governance.
Why do you need a data governance framework? 5 benefits
Let's talk about why this matters in practical terms. Poor data quality isn't just annoying—it's expensive. Research shows that bad data costs organizations an average of $12.9 million annually. But the impact goes well beyond just money as you’ll see below.
Improved data quality and consistency
You’ve probably experienced the frustration of making decisions based on data you're not quite sure about. Maybe you're looking at two different reports showing different numbers for the same metric. Or perhaps you're trying to analyze customer behavior but don't trust that your data is complete. A solid governance framework eliminates these uncertainties by establishing clear standards for data quality and maintaining consistency across all departments.
Enhanced regulatory compliance
Staying compliant in today’s complex regulatory environment isn't optional, it’s essential for survival. A well-structured governance framework makes compliance manageable by establishing clear protocols for data handling, privacy protection, and regulatory reporting. But it's not just about having these protocols, it's about keeping them current. Your framework should help you stay ahead of changing regulations and adapt your practices as new requirements emerge.
Stronger data security and risk management
Data breaches and security incidents can devastate an organization's reputation and bottom line. A robust governance framework helps you spot and address risks before they become problems through regular monitoring and security checks. This means implementing appropriate security measures (such as encryption, access controls, and data masking) and making sure everyone knows how to handle sensitive data safely.
Better decision-making with accurate data
When your data is reliable and well-governed, analytics become more powerful and decision-making becomes faster. No more second-guessing numbers or waiting for validation from multiple sources. Leaders can focus on strategic decisions rather than questioning the accuracy of their data.
Increased operational efficiency
A well-implemented data governance framework transforms how your organization handles data. When everyone follows the same processes and standards, you start seeing real improvements in data quality. Reports become more reliable. Decision-making gets faster because people trust the numbers they're looking at. And importantly, you spend less time double-checking data and more time actually using it to improve your business.
What are the four pillars of data governance?
Successfully managing your organization's data rests on four fundamental pillars. Understanding these helps you build a framework that actually works for your business.
1. Data stewardship
This entails making sure someone is actually responsible for taking care of your data. Good data stewardship means having people who understand both the technical aspects of data management and the business context of how that data gets used. These stewards become the go-to people when questions arise about data quality, access, or usage.
2. Data quality
Data quality forms the second pillar, and it's probably the one that most directly affects your day-to-day operations. We're talking about making sure your data is accurate, complete, and up-to-date. This means setting clear standards for what "good" data looks like in your organization and having processes to maintain those standards.
3. Data security
Security has become increasingly critical in recent years. With data breaches making headlines regularly, organizations can't afford to be casual about data security. This pillar involves everything from controlling who can access what data to protecting against external threats. Generally, it's about finding the right balance between making data accessible to those who need it while keeping it secure from those who shouldn't have access.
4. Data compliance
Compliance rounds out the four data governance pillars, and it's becoming more complex every year. With regulations like GDPR, CCPA, and industry-specific requirements, organizations need systematic ways to ensure they're following all applicable rules. A good compliance framework doesn't just help you avoid fines; it also helps build trust with your customers and partners by showing you take their data privacy seriously.
Data governance framework best practices
1. Establish ownership and accountability
Leadership buy-in is absolutely essential. Without support from the top, governance initiatives tend to fizzle out. This doesn't mean your CEO needs to understand every technical detail, but they do need to understand the value and actively support the initiative. Beyond executive sponsorship, establish clear ownership of data assets and create accountability frameworks that define who is responsible for what data and how they'll be held accountable for its quality and security.
2. Define policies and procedures
Starting small but thinking big is crucial. Organizations often fail when they try to transform everything at once. Instead, successful implementations typically begin with a single department or data type. For example, you might start with customer data in your sales department. This allows you to prove the value of governance on a smaller scale before rolling it out more broadly. As you scale, document clear policies and procedures for data handling, quality standards, and access controls. These procedures should be specific enough to guide action but flexible enough to adapt as your organization grows.
3. Implement automation solutions
Look for opportunities to automate routine governance tasks like data quality checks, access reviews, and policy compliance monitoring. Then choose automation solutions that integrate well with your existing tools and can scale with your governance program. Technology should support your governance efforts, not drive them. Too many organizations make the mistake of thinking they can solve their governance challenges just by buying new software. While good data governance tools are important, they need to support well-thought-out processes and clear organizational structures.
4. Maintain communication channels
Clear communication makes or breaks governance efforts. You need to explain what you're doing and why it matters in terms that resonate with different parts of your organization. Your sales team needs to understand how better data governance will help them close deals faster. Your finance team needs to see how it will make reporting more accurate and efficient. Regular audits and reviews ensure these communication channels stay open and effective. Establish formal channels for feedback, questions, and governance-related discussions, and create a regular cadence of updates to keep stakeholders informed of progress and changes.
3 data governance framework examples
Here are three widely-used frameworks that show how different organizations approach data governance:
McKinsey data governance model
McKinsey treats data governance as a business problem rather than just an IT issue. Their framework starts with getting top leaders involved—not just for show, but to actually drive the program. They suggest starting small by picking 2-3 critical data areas (like customer data or sales data) and focusing on those first. What makes their approach different is how they adjust the level of governance based on the company's needs. A global bank might need strict controls on everything, while a regional retailer can use a lighter touch for most of their data.
DGI data governance framework
The DGI framework is built around a simple idea: before you start managing data, you need to agree on how decisions about data will be made. They split governance into two parts. The first deals with practical things like creating data catalogs and setting quality standards. The second focuses on bigger questions like who gets to make decisions about data and what rules everyone needs to follow. A central office coordinates everything, but the actual work happens through councils and data stewards spread throughout the company.
PwC enterprise data governance framework
PwC breaks down data governance into clear, manageable pieces. Their framework looks at how data moves through an organization—from when it's first collected to when it's no longer needed. They focus heavily on making sure the right people are responsible for data at each step. What makes their approach useful is how it connects three things that often get handled separately: the people who work with data, the processes they follow, and the tools they use. This helps ensure that when you make changes to how data is governed, you're considering all three aspects.
These frameworks aren't meant to be followed exactly as most companies pick the parts that work best for their situation. The key is choosing an approach that matches how complex your data needs are and how strictly you need to control things.
Data governance framework template
1. Strategic overview
Component
Details
Vision
Define your organization's data vision
Mission
Outline specific goals and outcomes
Scope
List departments, systems, and data types covered
Timeline
Key milestones and implementation phases
Budget
Resource allocation and investment priorities
2. Organizational structure
Role
Responsibilities
Reports to
Executive sponsor
Overall accountability, strategic direction, and advocacy
Board
Data governance committee
Policy approval, oversight, and issue escalation
Executive sponsor
Chief data officer
Leads data governance program and aligns with business strategy
Executive sponsor
Data owners
Accountable for specific data domains and quality
Data governance committee
Data stewards
Day-to-day data management and issue resolution
Data owners
Data custodians
Technical implementation and system administration
IT director
Additional roles (e.g., data architects, analysts)
Specific responsibilities per organizational needs
Varies
3. Data classification
Category
Description
Security level
Retention period
Critical
Business-essential data
Highest
As needed
Sensitive
Regulated/private data
High
Per regulations
Confidential
Non-disclosure data
High
Per agreement
Internal
Operational data
Medium
Per policy
Public
Shareable data
Low
Unlimited
Additional categories
Per organizational needs
Varies
Varies
4. Core processes
Process
Owner
Frequency
KPIs
Quality monitoring
Data stewards
Weekly/Monthly
Error rates, completeness, timeliness
Access reviews
Security team
Monthly/Quarterly
Compliance %, unauthorized access attempts
Data audits
Governance committee
Quarterly/Annually
Coverage %, issue resolution time
Training
HR/Data office
Bi-annual/Annual
Completion rates, assessment scores
Metadata management
Data architects
Ongoing
Completeness, accuracy, standards compliance
Data integration
IT/Data engineers
Per project
Success rates, data quality, time-to-value
Archival & Retention
Data owners
Annually
Compliance %, storage costs
Incident management
Data stewards
As needed
Resolution time, impact assessment
Additional processes
Per organizational needs
Varies
Varies
5. Implementation checklist
- Assess current state and identify gaps
- Define and prioritize use cases
- Establish governance committee and assign roles
- Develop policies, standards, and procedures
- Define data domains and assign owners
- Create data dictionary and data lineage documentation
- Design data architecture and integration plan
- Select and implement data governance tools for cataloging, quality, security, etc.
- Establish access controls and monitoring processes
- Train staff on policies and procedures
- Measure and report on KPIs and business value
- Conduct regular reviews and maturity assessments
- Additional steps per organizational needs
This template provides a foundation that you can customize based on your organization's needs, size, and data complexity. Start with the most critical elements and expand the framework as your data governance program matures.
Achieve data compliance and security with Rippling
Implementing a data governance framework is a significant undertaking. It requires dedicated resources, specialized tools, and ongoing effort. That's where a platform like Rippling can be a significant asset.
Rippling is a unified workforce platform that helps organizations manage their HR, IT, and finance data in one place. But it's not just a data warehouse—it's designed with data governance in mind.
Rippling helps operationalize your data governance framework through:
- Role-based access controls: Manage data access permissions based on job functions, departments, and other attributes
- Identity & access management: Centralize user authentication and streamline access to company resources
- Dynamic MFA enforcement: Apply multi-factor authentication based on security requirements and user roles
- Automated deprovisioning: Instantly revoke access when employees leave or change roles
- Device management: Track and secure company devices with encryption and remote wipe capabilities
- Compliance certifications: Meet requirements for SOC 2 Type II, ISO 27001, and CSA STAR Level 2
- Audit logging: Track all system changes and access attempts for security monitoring
- Custom security policies: Create and enforce policies based on your organization's specific needs
- Automated workflows: Set up approval processes and security checks that run automatically
- Integration management: Control and monitor data flows between connected applications
In essence, Rippling can serve as the technological foundation of your data governance framework. It provides the tools and automations needed to put your policies into practice, without adding undue burden on your teams.
The platform's unified approach means you can manage all aspects of data governance—from access controls to compliance reporting—through a single interface, making it easier to maintain consistency and respond quickly to changing requirements.
Data governance framework FAQs
What are the most common data governance frameworks?
The most widely adopted frameworks include DAMA-DMBOK, DGI, PwC's enterprise framework, and McKinsey's model. Each offers different strengths: DAMA-DMBOK provides comprehensive coverage of all data management aspects, DGI focuses on decision-making processes, PwC emphasizes lifecycle management, and McKinsey takes a business-value approach. Organizations typically choose based on their industry, size, and specific needs.
What is the big data governance framework?
Big data governance frameworks extend traditional governance principles to handle the volume, variety, and velocity of big data. They're designed to manage both structured data (like spreadsheets) and unstructured data (like social media posts or sensor readings) flowing in from various sources—cloud services, connected devices, and applications. While there isn't a single standard 'big data governance framework,' organizations adapt their existing frameworks to handle these unique challenges while maintaining control over quality, security, and privacy at scale.
Is GDPR a data governance framework?
No, GDPR (general data protection regulation) is not a data governance framework, but rather a regulatory requirement that your data governance framework should address. While GDPR mandates specific data protection and privacy requirements, a data governance framework is broader and provides the structure for managing all aspects of data, including but not limited to compliance with regulations like GDPR, CCPA, and other privacy laws.
This blog is based on information available to Rippling as of February 7, 2025.
Disclaimer: Rippling and its affiliates do not provide tax, accounting, or legal advice. This material has been prepared for informational purposes only, and is not intended to provide or be relied on for tax, accounting, or legal advice. You should consult your own tax, accounting, and legal advisors before engaging in any related activities or transactions.