Meet Talent Signal: AI to help new hires succeed
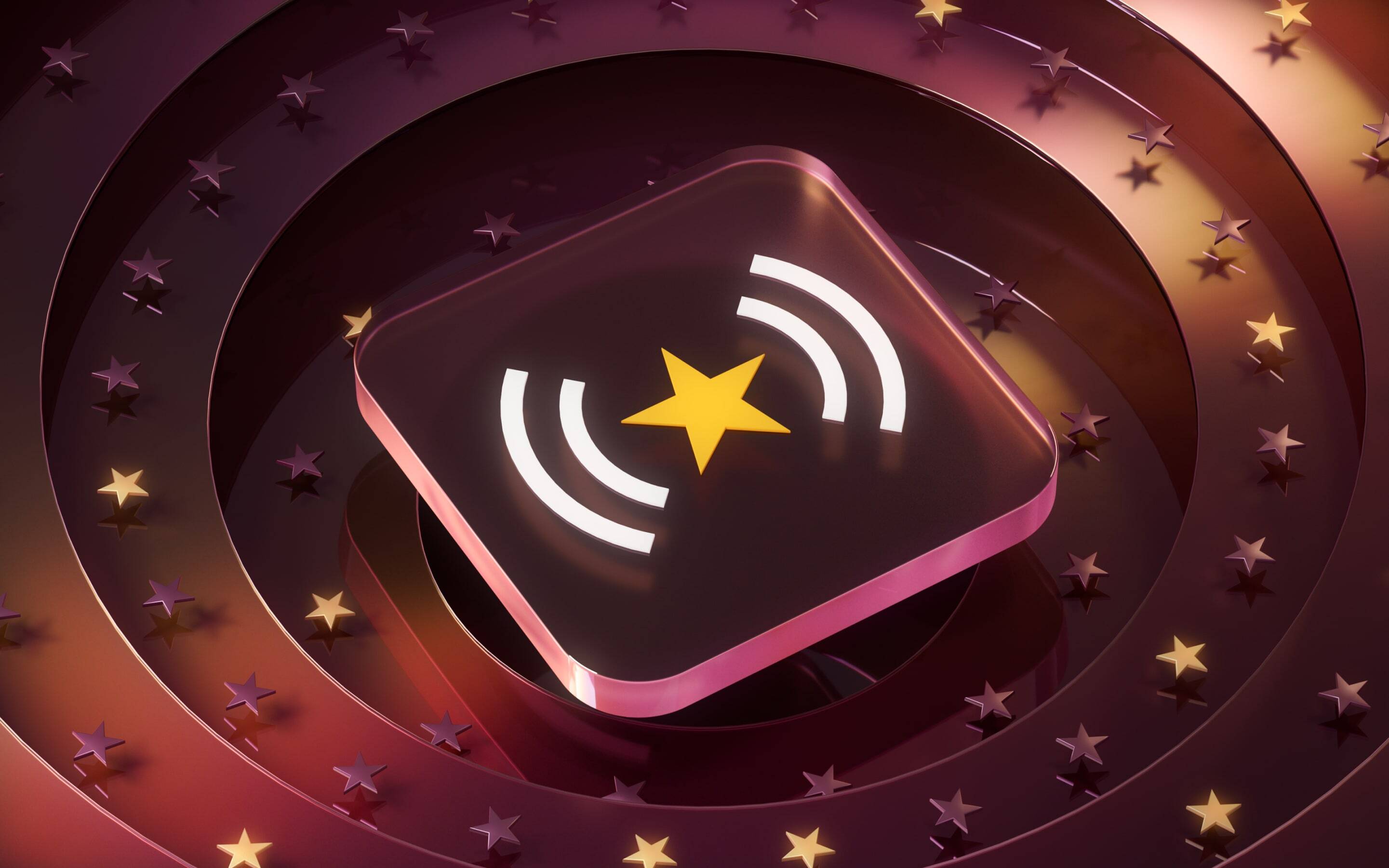
What if you could personally pull up a chair next to every new hire to review what they’ve done in their first 90 days, assess how they’re tracking, and provide helpful feedback? No manager has the time to do this, but we’ve built an AI tool that does. It’s called Talent Signal, and we’re inviting Rippling customers to help us refine it through an Early Access program, starting today.
Talent Signal reviews the first 90 days of work product for new hires in engineering, support, and, soon, sales to generate a performance signal for each employee. It provides an independent view of how a new employee is ramping, and gives managers concrete insights that they can use to coach them.
A useful signal, 90 days in
Most companies don’t have a solid understanding of how a new hire is performing until nearly a year into that employee’s tenure¹. In the meantime, those employees often don’t know where they stand. In cases where someone’s excelling, that’s a missed opportunity, and if they’re not meeting expectations, everyone involved suffers. Talent Signal helps by giving managers useful insights 90 days in, directing them toward fast-tracking their highest potential hires and coaching those who need support.
The magic of Talent Signal lies in its ability to reason directly from the work product. (For engineers, it’s reading their source code contributions. For your sales team, call transcripts. And for your support agents, customer emails and chats.) The model processes all of this work product data to gauge how well the employee is ramping up. It shares specific examples with the manager to allow for scrutiny of its conclusions.
When someone is newly onboarded, it’s one of the most sensitive and critical times. Having Talent Signal there at the beginning to provide directional information from 90 days worth of data lets us calibrate new team members and see how best to help them.
Keith Soura
CTO @ VERO
Of course, Talent Signal will never replace a manager in assessing the employee holistically, but it does provide an independent, fact-based, bottom-up assessment to give managers new information in assessing and supporting their team members.
Transparent, detailed reports
Talent Signal shows its work. It surfaces individual examples that support its signals, and it considers a wide range of relevant dimensions, such as code complexity for engineers and clarity of communications for support agents.
But Talent Signal isn’t just a crude scoring rubric. It’s a sophisticated model that synthesizes conclusions from all of the work product it can read for a given employee, scanning it for useful patterns that managers might miss, and surfacing the best for each employee. When an employee receives a “High Potential” or “Pay Attention” signal, it creates a detailed report for their manager, complete with links to the examples in the source system (e.g., Github, Salesforce, Zendesk) to support productive conversations between managers and employees.
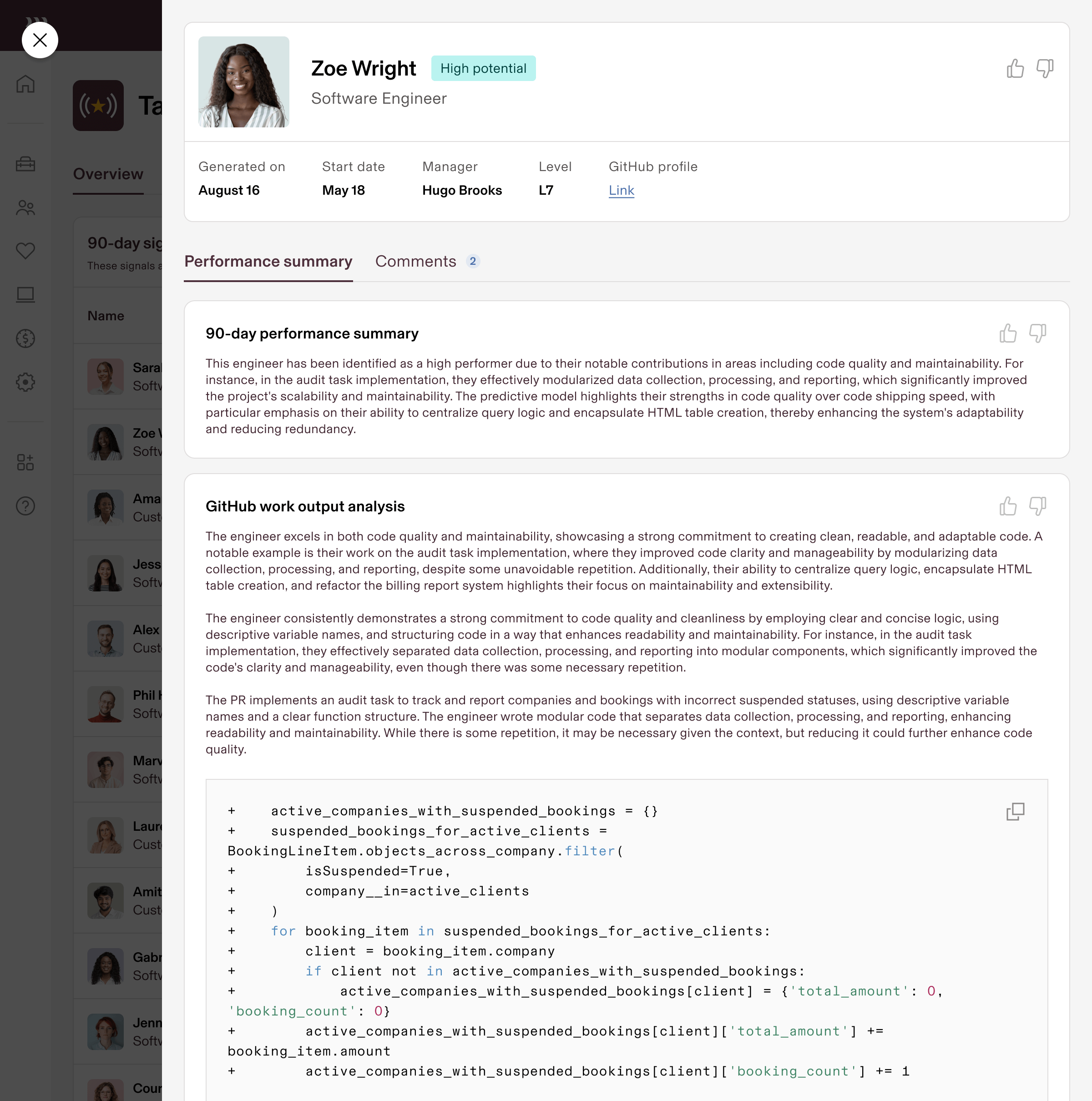
See employees for what they do
All too often, the assessment of an employee’s performance comes down to the subjective judgment of a single manager. During performance review cycles, managers and leadership try to reduce bias by performing a calibration across employees. Typically, the goal is to ensure that employees are evaluated consistently using a common set of standards. This approach is always imperfect, but it’s better than doing nothing at all.
When you add Talent Signal to the mix, new hires have a greater opportunity to be seen for what they do, rather than the perception of who they are. And because it offers a consistent, standardized input to reviewing the trajectory of new hires, quiet managers will be able to represent their team members as effectively as the loud ones. As with any input, a human must still judge the quality of the signal, but it’s likely the only input to the process that will be based solely on the employee’s work product.
Talent Signal is a tool to get another viewpoint. It’s a useful forcing function to get an orthogonal assessment on the quality of people's code. We use this as more of a tool to check our biases and ask ‘okay, are we missing something?’
August Allen
CTO of Enveda Biosciences
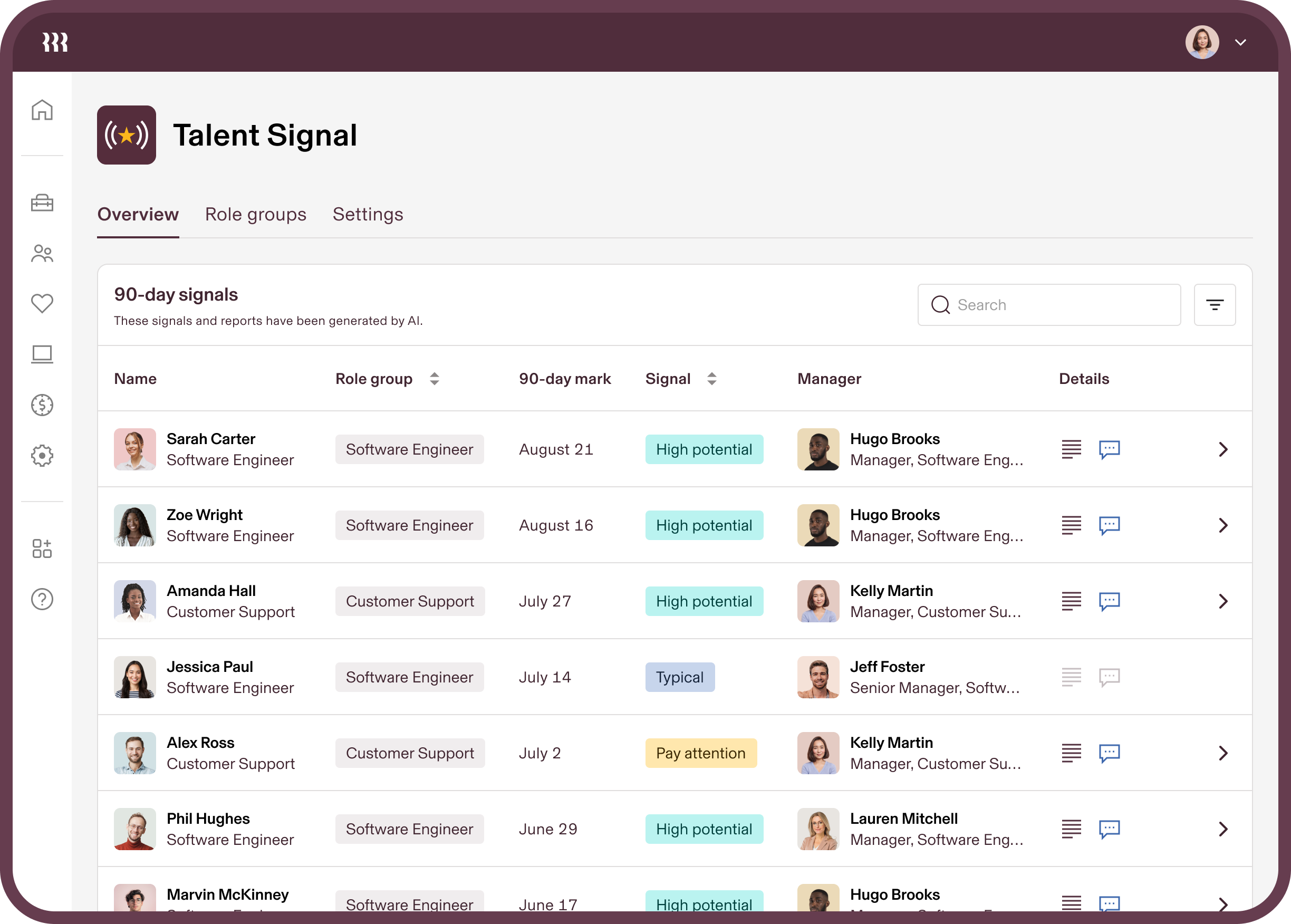
Responsible AI
The Early Access release of Talent Signal is trained on data from our own workforce as well as a group of alpha-test customers who opted in during its initial development, representing thousands of employees. Although results are promising, this Early Access program will allow us to further improve the model with a broader data set and additional direct feedback from customers. While the potential broader applications of Talent Signal are exciting, restricting its use to the first 90 days of an employee’s tenure allows us to refine it around a single employee milestone before applying it to additional use cases.
Artificial intelligence offers the promise of an exciting new future of work, and we view Talent Signal as our first step on that journey, with customers and other stakeholders guiding us along the way.
Help us build the future of AI at work
Join the Talent Signal waitlist below to be one of the first organizations to benefit from this new technology, and improve the success of new hires on your team.
¹ Survey methodology: The survey includes 169 managers and executives who are employed on a full-time basis at US-based companies with ≥50 employees.
Disclaimer: The performance signals and explanations produced by Talent Signal are intended to support managers and other leaders in existing human resources processes. It does not replace human decision-making.